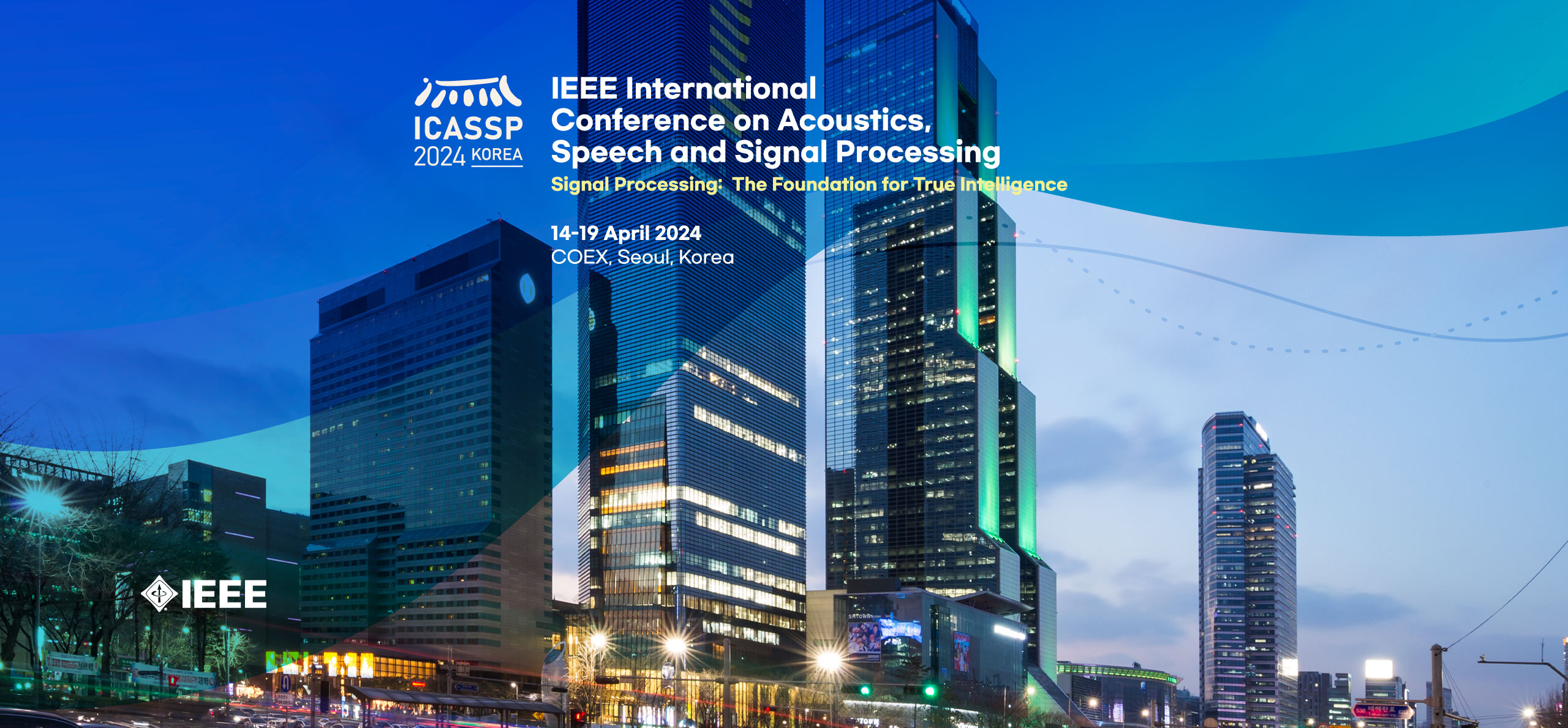
Timely and Private Machine Learning over Networks
Machine learning over networked systems, e.g., distributed/federated learning, is envisioned as the bedrock of future intelligent Internet-of-Things. By exploiting the computing power of end-user devices and inter-node communications, agents can exchange information with each other to collaboratively train a statistical model without centralizing their private data, which also contributes to the development of trustworthy intelligent systems. Despite its great potential, several new challenges must be addressed to make this paradigm possible. Specifically, in many applications, the parameters/states to be learned at different agents vary over time. And owing to impacts from data processing time, communication bandwidth, and transmission errors, the parameters delivered from one agent to the others may not be fresh. On the one hand, the stalled information impedes the performance of a distributed learning system, especially for real-time applications. On the other hand, the corrupted and stalled information improves end-users’ privacy, as instantaneous, accurate information is inaccessible. To that end, this workshop aims to foster discussion, discovery, and dissemination of novel ideas and approaches in the interplay between timeliness and privacy in machine learning over networks. We solicit high-quality original papers on topics including, but not limited to:
- Robust distributed learning algorithms against staleness in information exchange
- Privacy enhancing schemes (e.g., adopting differential privacy or creating synthetic data) for distributed learning systems
- Timeliness-aware distributed algorithms for networked machine learning systems
- Fundamental limits of network parameters on the performance of distributed learning systems
- Networking protocols to improve timeliness and privacy in distributed learning
- Over-the-air computation for private distributed machine learning systems
- Impact of network topology on the timeliness of distributed machine learning algorithms
- Robust and Private distributed reinforcement/meta/deep learning and other novel learning paradigms
- Novel methods for distributed machine learning with limited communication resources
- Experimental implementations and testbeds on large-scale distributed learning systems
Workshop Organizers
- Howard H. Yang, ZJU-UIUC Institute, Zhejiang University, China
- Nikolaos Pappas, Department of CIS, Linköping University, Sweden
- Harpreet S. Dhillon, Bradley Department of Electrical and Computer Engineering, Virginia Tech
Schedule
Sunday, April 14th, 2024
08:30 — 12:00 South Korea Time
Location: Room 209A
Session 1 (Session chair: Prof. Nikolaos Pappas)
08:30 - 08:45 am
Opening Remarks
08:45 - 09:00 am
Towards Efficient Backdoor Attacks Against Federated Self-Supervised Learning Through Intra-Union Aggregation
09:00 - 09:15 am
Convergence Analysis of Semi-Federated Learning with Non-IID Data
09:15 - 09:30 am
A Compressed Decentralized Federated Learning Framework for Enhanced Environmental Awareness in V2V Networks
09:30 - 09:45 am
Over the Air Federated Learning in the Presence of Impulsive Noise
09:45 - 10:15 am
Coffee Break
Session 2 (Session chair: Prof. Nikolaos Pappas)
10:15 - 10:30 am
FedRF-Adapt: Robust and Communication-Efficient Federated Domain Adaptation via Random Features
10:30 - 10:45 am
Recommendation Algorithm Based on Federated Multi-modal Learning
10:45 - 11:00 am
Version Age-Based Client Scheduling Policy for Federated Learning
11:00 - 11:15 am
PerSOTA FL: A Robust-to-Noise Personalized Over the Air Federated Learning for Human Activity Recognition
11:15 - 11:30 am
Towards Collaborative Multi-modal Federated Learning for Human Activity Recognition in Smart Workplace Environments
11:30 - 11:45 am
Byzantine-Resilient Hierarchical Federated Learning with Clustered Over-the-Air Aggregation
Submission Guidelines
Manuscripts should conform to the ICASSP paper format as stated in the
main conference.
Important Dates
Workshop Paper Submission Deadline: December 31, 2023 (23:59 AoE)
Workshop Paper Acceptance Notification: January 31, 2024 (23:59 AoE)
Workshop Camera Ready Paper Deadline: February 10, 2024 (23:59 AoE)
Paper Submission Link
Workshop papers can be submitted via the following link:
Paper_submission_link